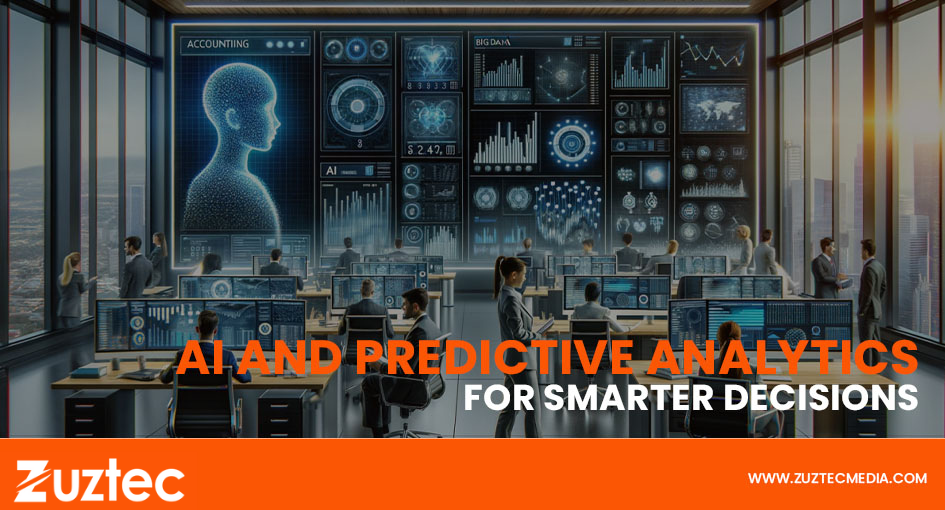
AI And Predictive Analytics for Smarter Decisions
In today’s data-driven world, businesses are constantly seeking ways to make smarter, more informed decisions. AI-powered predictive analytics has emerged as a powerful tool that allows companies to do just that. By combining artificial intelligence (AI) with advanced data analysis, predictive analytics enables businesses to forecast future trends, identify potential risks, and make proactive decisions based on data-driven insights.
AI and predictive analytics goes beyond traditional data analysis by leveraging machine learning algorithms to analyze vast amounts of historical and real-time data. These algorithms can detect patterns, correlations, and anomalies that might be missed by human analysts, leading to more accurate predictions and actionable insights. From sales forecasting and customer behavior analysis to risk management and operational efficiency, AI-driven predictive analytics is transforming how businesses operate.
This technology is particularly valuable for industries like finance, healthcare, retail, and manufacturing, where accurate forecasting can significantly impact decision-making and outcomes. For example, financial institutions can use predictive analytics to detect fraudulent activities, while retailers can optimize inventory by predicting consumer demand.
AI-powered predictive analytics can improve decision-making, reduce risks, and unlock growth opportunities for businesses. As AI evolves, its potential for smarter, more effective decisions will grow.
How AI And Predictive Analytics Works
Predictive analytics uses historical and current data to predict future events, with AI-powered methods enhancing this process by incorporating machine learning algorithms and techniques to refine predictions over time.
Here’s a breakdown of how AI-powered predictive analytics functions:
- Data Collection and Preparation: AI and predictive analytics involves gathering and preparing data from various sources, including customer transactions, social media interactions, and financial reports, using AI systems to leverage relevant information.
- Feature Engineering: AI algorithms require meaningful inputs to make accurate predictions. In this step, analysts select and transform relevant features (variables) from the raw data that will be used to train the predictive models. This step is crucial in ensuring that the models can effectively identify patterns and relationships within the data.
- Model Training and Learning: Machine learning models identify patterns and trends using selected data, learning from past events and making predictions based on new information, continuously refining their accuracy over time.
- Prediction and Interpretation: AI-powered models can predict future outcomes, identify customer behavior, and predict sales, while also providing explanations and understanding of trends through interpretation.
- Continuous Improvement: AI and predictive analytics models, unlike traditional models that require manual updates, can improve automatically as more data is collected and analyzed, providing businesses with more reliable insights.
Applications of AI-Powered Predictive Analytics Across Industries
AI-powered predictive analytics is widely used across various industries for smarter decision-making, growth, and operational efficiency improvement, with key applications across various sectors.
- Finance and Banking: Predictive analytics in the financial sector aids in risk assessment, market trend forecasting, fraud prevention, informed decision-making, investment portfolio optimization, and personalized customer service.
- Retail and E-commerce: AI-powered predictive analytics aids retailers and e-commerce businesses in forecasting demand, optimizing inventory management, and enhancing customer experiences by analyzing consumer behavior and purchasing history.
- Healthcare: Predictive analytics is revolutionizing healthcare by enabling early diagnosis, personalized treatment plans, and predictive maintenance of medical equipment, enabling AI-powered systems to identify risk factors and efficiently manage resources.
- Manufacturing: Predictive analytics in manufacturing optimizes production, reduces downtime, and prevents equipment failures by analyzing sensor data, scheduling maintenance, and minimizing maintenance costs.
- Marketing and Advertising: AI-powered predictive analytics aids marketing strategies by analyzing customer data to target the right customers at the right time, maximizing ROI and improving customer engagement, thus enhancing marketing campaigns and advertising effectiveness.
- Supply Chain and Logistics:AI and Predictive analytics optimizes logistics and supply chain operations by forecasting demand, managing inventory, and reducing transportation costs, resulting in faster deliveries, lower costs, and improved customer satisfaction.
Benefits of AI-Powered Predictive Analytics
The integration of AI predictive analytics offers several key advantages for businesses seeking to make smarter decisions:
- Improved Accuracy: AI-powered models are capable of processing vast amounts of data and identifying patterns that are difficult for humans to detect. As a result, forecasts become more precise, helping firms to make more informed decisions.
- Efficiency and Automation: AI-powered predictive analytics automates data analysis, reducing time and effort required for insights, enabling businesses to swiftly respond to market changes and customer needs.
- Cost Reduction:AI Predictive analytics optimizes processes, improves resource allocation, and minimizes risks, reducing costs in manufacturing and retail sectors like manufacturing and retail.
- Personalization: AI-powered predictive analytics enables businesses to provide personalized customer experiences by analyzing individual preferences, thereby enhancing satisfaction and loyalty.
- Risk Mitigation: Predictive analytics aids businesses in identifying potential risks, thereby enabling proactive measures to mitigate them, especially in sectors like finance and healthcare.
Challenges and Considerations
While AI-powered predictive analytics offers numerous benefits, it also comes with challenges that businesses must address:
- Data Quality and Availability: Predictive analytics accuracy relies on data quality and quantity, and businesses must invest in proper data collection and management practices for reliable outcomes.
- Integration with Existing Systems:AI-powered predictive analytics implementation necessitates complex integration with existing business systems, necessitating infrastructure updates and staff training for efficient use.
- Ethical Considerations: AI’s use in decision-making raises ethical concerns, including data privacy and algorithm bias, necessitating businesses to ensure transparency, fairness, and regulatory compliance.
Conclusion, AI and predictive analytics is transforming the way businesses operate, offering them the ability to make smarter, data-driven decisions. From finance and healthcare to retail and manufacturing, the applications of this technology are vast and varied. By leveraging AI to analyze data, predict trends, and optimize operations, businesses can improve efficiency, reduce costs, and enhance customer experiences.However, to fully realize the potential of AI-powered predictive analytics, businesses must address challenges such as data quality, system integration, and ethical considerations. With the right approach, AI-powered predictive analytics can unlock new levels of innovation and drive smarter decision-making in today’s rapidly evolving marketplace.