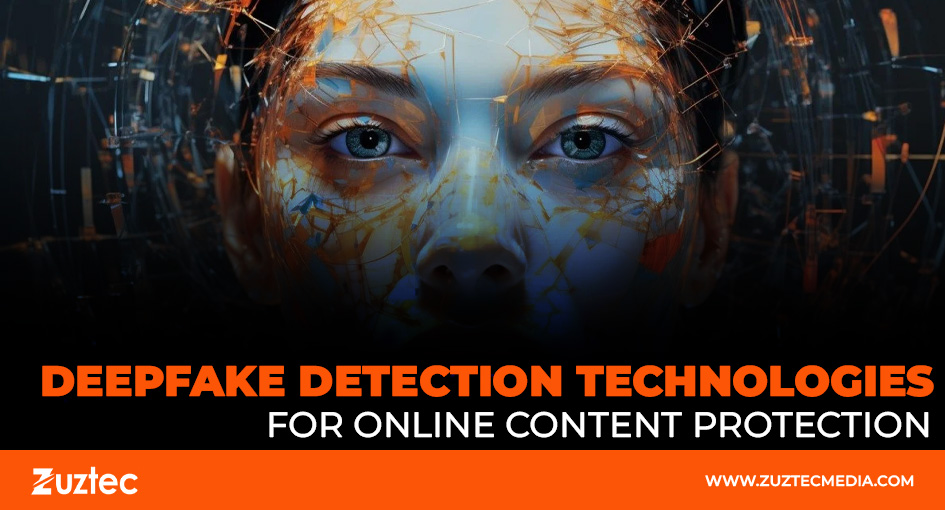
Deepfake Detection Technologies for Online Content Protection
Deepfakes are becoming a growing concern in the digital world. These are fake videos, images, or audio clips created using artificial intelligence to make someone appear to say or do something they never actually did. At first glance, deepfakes can look extremely real, which is why they’re so dangerous. The abuse of deepfakes poses a major risk to national security, media credibility, and individual privacy by disseminating misleading information and destroying reputations.
As deepfake content spreads rapidly across social media and online platforms, the need for reliable detection has become urgent. These tools and methods are designed to analyze content and identify signs that it may have been artificially generated or altered. The technology works by spotting inconsistencies that humans can’t easily detect, such as irregular blinking patterns, unnatural skin textures, or mismatched audio and video cues.
Various industries now rely on deepfake detection to protect against fraud and misinformation. News outlets use it to verify the authenticity of videos before publishing. Social media platforms employ it to flag manipulated content. Even law enforcement uses these tools during investigations to determine the credibility of video evidence.
This article explores how deepfake detection technologies work, the tools available today, and the challenges involved. As deepfake creation tools become more advanced, detection technologies must evolve alongside them. Understanding these technologies is crucial in building a safer and more trustworthy digital environment.
Understanding How Deepfakes Are Created
Before diving into detection methods, it’s helpful to understand how deepfakes are made. Deepfakes are typically created using machine learning techniques, especially deep learning and generative adversarial networks (GANs). A GAN consists of two neural networks—one that generates fake content and one that tries to detect if it’s fake. Through a series of adjustments, the generator becomes better at creating believable fakes.
These tools can swap faces in videos, create synthetic voices, or even generate entirely new visual identities. With enough data, even an amateur can now make a convincing deepfake using open-source software. The low cost and wide availability of these tools make deepfakes a widespread threat.
How Deepfake Detection Technologies Work
They use artificial intelligence to find patterns and flaws that indicate manipulation. Unlike the human eye, AI can analyze pixel-level data and frame-by-frame inconsistencies.
Here are some of the methods these tools use:
- Facial Movement Analysis: AI looks at blinking rates, mouth movement, and facial expressions. Many early deepfakes failed to blink properly, revealing their fake nature.
- Texture and Lighting Checks: Deepfakes often show irregular lighting, blurring around facial edges, or inconsistent skin tones. Detection software can scan for these anomalies.
- Audio-Video Synchronization: Some tools check if the speaker’s voice matches lip movements. A mismatch often signals fake content.
- Biometric Features: Detection tools examine micro-expressions and other subtle cues that are hard for fakes to replicate.
- Source Verification: Some technologies cross-reference videos or images with databases of verified content to see if anything has been altered.
Popular Deepfake Detection Technologies Tools
- Microsoft Video Authenticator: Microsoft developed this tool to analyze photos and videos and provide a confidence score showing how likely it is that the content is a deepfake.
- Deepware Scanner: Deepware offers a browser-based tool that allows users to upload videos and scan them for signs of manipulation. It’s used by journalists, researchers, and security agencies.
- FaceForensics++: A database used for training and testing deepfake detection algorithms. It helps researchers evaluate how well their tools perform under different conditions.
- Intel FakeCatcher: This real-time detection tool analyzes blood flow in the human face, which is nearly impossible to fake convincingly. It’s highly effective against even advanced deepfakes.
Applications Across Different Industries
- Media and Journalism: News agencies use detection tools to verify content before publishing. This prevents the spread of misinformation and maintains trust with audiences.
- Social Media Platforms: Companies like Facebook and TikTok are investing in deepfake detection to automatically identify and remove harmful content before it spreads.
- Cybersecurity: Detection tools help organizations prevent social engineering attacks that use deepfakes for phishing or identity fraud.
- Law Enforcement: Video and audio evidence is increasingly checked using AI tools to ensure it hasn’t been altered or created by deepfake software.
- Education and Awareness: Schools and universities are also incorporating these technologies into media literacy programs to help students recognize manipulated content.
Consequently, deepfake detection technologies are now essential tools for maintaining trust and safety in the digital world. As deepfake creation becomes more realistic and accessible, the risks to public figures, private individuals, and institutions increase. By leveraging advanced detection tools and adopting best practices, we can better protect against misinformation and digital fraud. Staying informed and proactive is the best defense in the evolving battle against deepfakes.